Seasonal-to-decadal prediction
An area of climate prediction with the most immediate socio-economic applications (e.g. in the areas of agriculture, energy strategy, water supply, coastal protection, insurance) is that of seasonal-to-decadal forecasting. A major challenge in climate research is to develop skilful capability on these timescales and with useful spatial resolution. Representing the influence of the oceans is vital since they have a much larger capacity to store heat and freshwater than the atmosphere and also have much slower modes of propagating climate anomalies. These factors result in the oceans having a much longer “memory” in the climate system and thus the potential for longer forecast lead times when models are initialised with an estimate of ocean state.
Argo is essential for providing the sub-surface observations required to initialise and validate both seasonal (Balmaseda et al., 2007) and decadal climate forecasts (Smith et al., 2007). The delivery of both collocated temperature and salinity is required to gain skill in forecasting the time evolution of the climate system, including the meridional overturning circulation (MOC, e.g. Bryden et al., 2005), which is the focus of the NERC RAPID program. Model studies suggest that a collapse of the MOC due to additional freshwater input associated with global warming could cause a rapid regional temperature change of -2°C over Western Europe (Vellinga and Wood, 2002). Below is a case study on decadal forecasts of the MOC using simulated Argo observations in the Met Office decadal prediction system (Smith et al., 2007).
Impact of Argo on forecasts of the meridional overturning circulation
Western Europe’s mild climate is sustained by high poleward heat transport by the global meridional overturning circulation (MOC). The large and rapid transitions between glacial and interglacial periods have been linked to changes in the MOC and in the location and intensity of deep winter convection at high latitudes. Model studies suggest that a collapse of the MOC due to addition of freshwater under global warming could cause a regional temperature change of -2°C over Western Europe (Vellinga and Wood, 2002). The NERC RAPID programme is studying the stability and variability of the MOC. The fact that Argo measures both salinity and temperature (and therefore density can be derived) makes the data particularly valuable for understanding and predicting changes of the MOC (e.g. Bryden et al., 2005).
Validating decadal forecast systems, using “hindcasts”, is currently very difficult due to the extremely limited coverage prior to Argo. Idealised (or “Perfect model”) predictability studies enable us to cleanly separate the affects of assimilating different observations without having to take external forcings or observational uncertainty into account. As a basis for our experiments we use a long control run of the Hadley Centre Coupled Model, version 3 (HadCM3, Gordon et al., 2000). All our assimilation experiments start using model conditions taken from a distant part of the control run. We focus on four different assimilation schemes:
- ‘Perfect' - uses conditions from the original control run
- ‘Full-Depth' - ocean temperature (T) and salinity (S) are assimilated to full depth
- ‘2000m' - ocean T and S are assimilated to 2,000m (c.f. Argo)
- ‘SST Only' - sea surface temperature (SST) is assimilated
For the ’Full Depth‘ and ‘2000m‘ experiments we use the same, relatively strong, relaxation to assimilated data as in Smith et al. (2007). Similarly, both experiments assimilate six-hourly atmospheric data. In the case of the ‘SST Only’ experiment we follow the assimilation method of Keenlyside et al. (2008). This has a weaker and latitude dependent relaxation timescale and also does not assimilate the atmosphere. The assimilation runs provide the initial conditions for the forecasts.
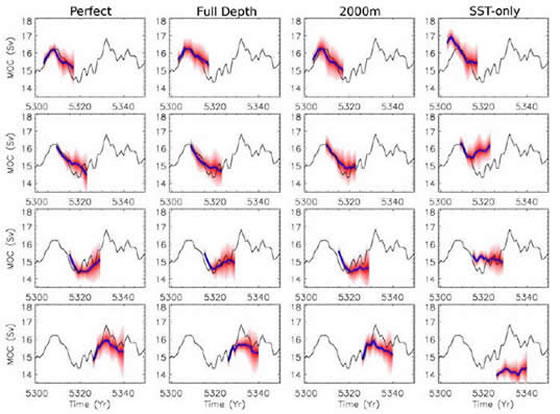
Forecasts of the MOC for the four experiments (each with different ocean initialisation) and for four start dates. The thick blue lines are the ensemble means and the red shading indicates the 90% confidence region.
The forecasts are nine-member initial condition perturbed ensembles and are run for 15 years. In the above figure we show the forecasts of the MOC for all experiments and four start dates which were chosen to sample a range of phases of the MOC. The Full depth and 2000m experiments show a similar level of skill to that of the Perfect experiment. This is particularly encouraging as it suggests that assimilating 2,000m T and S data (as provided by the Argo array) has the potential to provide skilful forecasts of the MOC. In contrast, when we only assimilate SST the model does a relatively poor job at reproducing the evolution of the original MOC.
In the figure below we look at the skill of forecasting SST. Here we correlate anomaly maps of five year mean forecast SST against the truth from each forecast start date. We calculate an average correlation from all start dates and then plot this as a function of forecast lead time for each of the four experiments. The figure clearly illustrates that the forecast skill is dependent upon the amount of information that we assimilate. The Perfect experiment, having full knowledge of all variables (including ocean currents), gives the highest skill at all lead times. Initially the Full depth and 2000m T and S experiments show only slightly less skill than the Perfect experiment. At longer lead times the skill of the 2000m experiment starts to decrease more rapidly than that of the Perfect and Full depth experiments. This suggests that initialising the deeper ocean is starting to have a surface impact after a couple of years. The skill of the SST Only experiment, while positive, is very much less than the experiments that assimilate sub-surface temperature and salinity data. This simple experiment illustrates the potential of Argo data to successfully initialise decadal climate models.
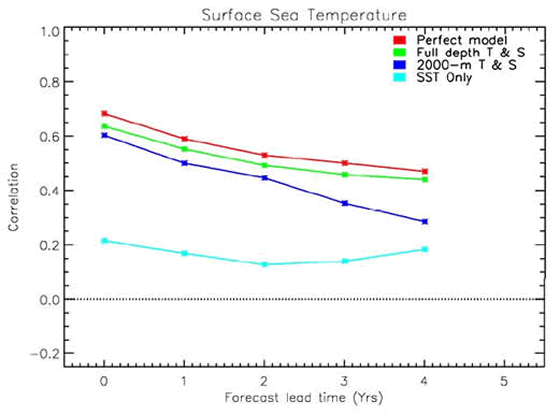
The skill in forecasting 5 year SST anomalies is shown for the four different experiments. Note that the skill in these idealised experiments depends upon the amount of information assimilated. The Argo like 2000m T & S experiment compares favourably to the Perfect and Full depth experiments, although skill does drop off quicker at longer lead times. The experiment that assimilates SST only shows relatively poor skill at all lead times.